4.0. Application of data analytics in specialised areas
4.2. Auditing
Carry out audit sampling from large data set
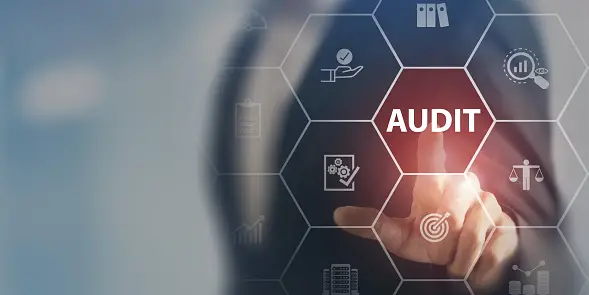
Application of Data Analytics in Auditing
Auditing in the context of a large data set often involves the use of audit sampling techniques to efficiently assess the data's integrity and compliance. Instead of examining every single transaction or record, which may be impractical due to the dataset's size, auditors employ statistical sampling methods to select a representative subset for analysis. This subset, chosen using various sampling approaches like random, stratified, or systematic sampling, allows auditors to draw conclusions about the entire dataset while minimizing time and resource expenditures. By carefully designing and executing an audit sampling plan, auditors can uncover potential errors, anomalies, or irregularities in the data, helping to ensure accuracy and compliance with relevant regulations and standards.
Related content:
4.2. | Auditing |
4.2.1. Analysis of trends in key financial statements components 4.2.2. Carry out 3-way order matching 4.2.3. Fraud detection 4.2.4. Test controls (specifically segregation of duties) by identifying combinations of users involved in processing transactions 4.2.5. Carry out audit sampling from large data set 4.2.6. Model review and validation issues
Revolutionizing Audit Sampling: Big Data's Impact on Efficiency and Insights
In the digital age, businesses generate vast amounts of data, making traditional audit sampling methods less practical for thorough assessments. Enter data analytics, a game-changer that leverages big data to revolutionize audit sampling processes. This article delves into the innovative techniques and tools that are reshaping auditing, enhancing efficiency, accuracy, and insights, while uncovering hidden risks and opportunities in financial records and compliance assessments.
The Challenge of Large Datasets:
Auditing has traditionally relied on random sampling, which can be time-consuming and may not reveal all anomalies or irregularities in extensive datasets. Large datasets can easily overwhelm auditors, leading to potentially missed issues that could have serious financial and legal implications. This is where data analytics steps in, offering a more systematic and comprehensive approach.
Data Analytics in Audit Sampling:
- Advanced Sampling Methods: Data analytics enables auditors to employ advanced sampling techniques such as stratified sampling, systematic sampling, and clustering, allowing for a more targeted and precise analysis of data subsets.
- Pattern Recognition: Data analytics tools can identify patterns and trends within the data, helping auditors pinpoint unusual transactions or deviations from expected norms. This is particularly useful in fraud detection and risk assessment.
- Machine Learning Algorithms: Machine learning algorithms can analyze historical data to predict potential risks or outliers in real-time, improving audit efficiency by prioritizing areas that require immediate attention.
- Continuous Monitoring: With data analytics, auditing becomes an ongoing process rather than a periodic event. Continuous monitoring of data allows auditors to detect irregularities as they occur, reducing the likelihood of long-standing issues.
Enhanced Efficiency:
By automating repetitive tasks and analyzing vast datasets quickly, data analytics significantly improves audit efficiency. Auditors can focus their efforts on high-risk areas, saving time and resources. This efficiency also translates into shorter audit cycles, benefiting both auditors and auditees.
Accuracy and Precision:
Data analytics minimizes the margin of error associated with manual sampling. It ensures that the selected samples are truly representative of the entire dataset, reducing the risk of overlooking critical issues. Auditors can be more confident in their findings and conclusions.
Insightful Analysis:
Beyond compliance, data analytics offers auditors the ability to derive valuable insights from financial records. Auditors can identify areas of operational improvement, potential cost savings, and revenue enhancement opportunities, turning audits into a strategic asset for businesses.
Auditing & Assurance
Table of contents
Syllabus
-
1.0
Introduction to Excel
- Microsoft excel key features
- Spreadsheet Interface
- Excel Formulas and Functions
- Data Analysis Tools
- keyboard shortcuts in Excel
- Conducting data analysis using data tables, pivot tables and other common functions
- Improving Financial Models with Advanced Formulas and Functions
-
2.0
Introduction to data analytics
-
3.0
Core application of data analytics
- Financial Accounting And Reporting
- Statement of Profit or Loss
- Statement of Financial Position
- Statement of Cash Flows
- Common Size Financial Statement
- Cross-Sectional Analysis
- Trend Analysis
- Analyse financial statements using ratios
- Graphs and Chats
- Prepare forecast financial statements under specified assumptions
- Carry out sensitivity analysis and scenario analysis on the forecast financial statements
- Data visualization and dash boards for reporting
- Financial Management
- Time value of money analysis for different types of cash flows
- Loan amortization schedules
- Project evaluation techniques using net present value - (NPV), internal rate of return (IRR)
- Carry out sensitivity analysis and scenario analysis in project evaluation
- Data visualisation and dashboards in financial management projects
4.0
Application of data analytics in specialised areas
- Management accounting
- Estimate cost of products (goods and services) using high-low and regression analysis method
- Estimate price, revenue and profit margins
- Carry out break-even analysis
- Budget preparation and analysis (including variances)
- Carry out sensitivity analysis and scenario analysis and prepare flexible budgets
- Auditing
- Analysis of trends in key financial statements components
- Carry out 3-way order matching
- Fraud detection
- Test controls (specifically segregation of duties) by identifying combinations of users involved in processing transactions
- Carry out audit sampling from large data set
- Model review and validation issues
- Taxation and public financial management
- Compute tax payable for individuals and companies
- Prepare wear and tear deduction schedules
- Analyse public sector financial statements using analytical tools
- Budget preparation and analysis (including variances)
- Analysis of both public debt and revenue in both county and national government
- Data visualisation and reporting in the public sector
5.0
Emerging issues in data analytics