4.0. Application of data analytics in specialised areas
4.2. Auditing
Fraud detection
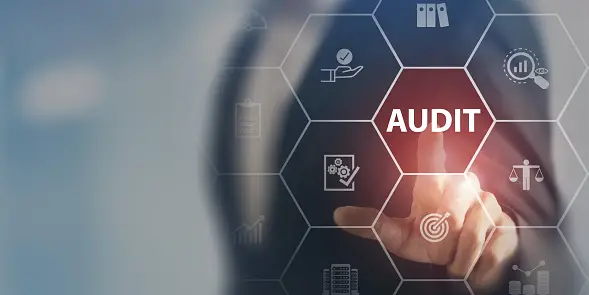
Application of Data Analytics in Auditing
What is fraud detection ?
Fraud detection is the process of identifying and preventing fraudulent activities or unauthorized actions within an organization or system. It involves the use of various techniques, technologies, and strategies to uncover deceptive or malicious behaviors that can result in financial losses, reputational damage, or legal consequences. Fraud detection is essential for safeguarding the integrity and security of businesses, financial institutions, government agencies, and other organizations.
Related content:
4.2. Auditing
4.2.1. Analysis of trends in key financial statements components 4.2.2. Carry out 3-way order matching 4.2.3. Fraud detection 4.2.4. Test controls (specifically segregation of duties) by identifying combinations of users involved in processing transactions 4.2.5. Carry out audit sampling from large data set 4.2.6. Model review and validation issues
Application of Data Analytics in fraud detection
Data analytics has emerged as a powerful tool in the realm of fraud detection, revolutionizing the way organizations identify and prevent fraudulent activities. With the increasing sophistication of fraudsters and the growing volume of data generated in today's digital world, data analytics provides an efficient and effective means to detect and combat various forms of fraud. Here's a detailed exploration of the application of data analytics in fraud detection:
1. Data Integration and Aggregation:
Data analytics begins with the collection and aggregation of vast datasets from various sources, including financial transactions, customer interactions, and system logs.
These datasets are integrated into a centralized repository, allowing for a comprehensive view of organizational data.
2. Anomaly Detection:
Anomaly detection is a fundamental aspect of fraud detection using data analytics. Machine learning algorithms and statistical techniques are applied to identify unusual patterns or outliers in the data.
Anomalies can be indicative of fraud, such as unusual transaction amounts, atypical user behavior, or deviations from historical trends.
3. Pattern Recognition:
Data analytics leverages historical data to recognize patterns associated with fraudulent activities.
By comparing current data to established patterns, analytics tools can identify deviations that may suggest fraudulent behavior.
For example, recognizing patterns of identity theft or fraudulent insurance claims becomes more feasible through pattern recognition.
4. Real-time Monitoring:
Many fraud detection systems operate in real-time or near-real-time, continuously monitoring transactions and activities as they occur.
Real-time monitoring allows for immediate detection and response to suspicious activities, reducing the potential impact of fraud.
5. Predictive Analytics:
Predictive analytics uses historical data and statistical modeling to forecast future fraudulent activities.
It helps organizations proactively implement preventive measures and allocate resources effectively to mitigate fraud risks.
6. Machine Learning and Artificial Intelligence (AI):
Machine learning and AI techniques, such as neural networks and deep learning, enable more advanced and adaptive fraud detection.
These technologies can uncover complex and evolving fraud schemes that may be challenging to detect using traditional methods.
7. Text and Natural Language Processing (NLP):
NLP can be applied to analyze unstructured text data, such as emails, chat logs, and social media posts, to identify potential fraud-related communications or narratives.
8. Visualization Tools:
Data visualization tools are used to represent complex data patterns in a visually understandable format.
Visualization aids in identifying trends, outliers, and anomalies that may require further investigation.
9. Collaboration with Subject Matter Experts:
Data analytics teams often collaborate with subject matter experts, including fraud investigators and forensic accountants, to refine algorithms and enhance fraud detection models.
10. Compliance and Regulatory Reporting:
Data analytics helps organizations adhere to regulatory requirements by monitoring transactions for compliance violations and generating reports for regulatory authorities.
Benefits of Data Analytics in Fraud Detection:
- Timely Detection: Data analytics allows for early detection of fraudulent activities, reducing financial losses and damage to reputation.
- Improved Accuracy: Automated analytics tools are less prone to human errors, ensuring more accurate fraud identification.
- Cost Savings: Identifying and preventing fraud early can lead to substantial cost savings for organizations.
- Adaptability: Data analytics can adapt to evolving fraud schemes, making it a valuable asset in the fight against increasingly sophisticated fraudsters.
Data analytics has become an indispensable component of modern fraud detection efforts. Its ability to analyze large datasets, detect anomalies, and recognize complex patterns empowers organizations to proactively combat various forms of fraud, safeguard their assets, and maintain trust with customers and stakeholders. As fraud schemes continue to evolve, data analytics will remain a critical tool in staying one step ahead of fraudsters.
Types of fraud and how Data Analytics can be used to detect and prevent them
Fraud is a pervasive threat that comes in various forms, targeting individuals, businesses, and organizations. From financial fraud to cybersecurity breaches, understanding the different types of fraud is crucial for effective prevention and mitigation. Data analytics has emerged as a powerful weapon in the fight against fraud, offering insights, automation, and real-time monitoring capabilities. Here's an exploration of common types of fraud and how data analytics can be employed to detect and prevent them:
Financial Fraud:
➧ Types: This encompasses a wide range of fraudulent activities, including accounting fraud, embezzlement, identity theft, and credit card fraud.
➢ Data Analytics Application*: Data analytics tools can scrutinize financial transactions for anomalies and patterns that deviate from the norm. For example, algorithms can detect unusual spending behavior on a credit card or unauthorized fund transfers.
Insurance Fraud:
➧ Types: Insurance fraud can involve fraudulent claims, staged accidents, or exaggeration of damages.
➢ Data Analytics Application: By analyzing historical claim data and comparing it to current claims, data analytics can identify patterns of behavior consistent with fraudulent claims. Predictive modeling can also forecast potential fraudulent activities.
Healthcare Fraud:
➧ Types : Healthcare fraud includes billing for services not provided, upcoding (charging for a more expensive service), and prescription fraud.
➢ Data Analytics Application: Data analytics can analyze healthcare billing data to identify outliers and anomalies, flagging providers or claims that require further investigation.
Cybersecurity Fraud:
➧ Types : Cyber fraud comprises various threats like hacking, phishing, malware attacks, and data breaches.
➢ Data Analytics Application: Data analytics tools monitor network traffic for unusual activities, such as unauthorized access attempts or data transfers. Machine learning models can detect patterns consistent with malware behavior.
Tax Evasion and Fraud:
➧ Types: This includes underreporting income, inflating deductions, or engaging in tax shelters to evade taxes.
➢ Data Analytics Application: Data analytics can analyze tax return data for inconsistencies or deviations from statistical norms, helping tax authorities identify potential fraud.
Procurement Fraud:
➧ Types: Procurement fraud involves corruption, kickbacks, bid rigging, or overpayment for goods and services.
➢ Data Analytics Application: By analyzing procurement data, data analytics can detect irregularities such as suspicious supplier relationships or pricing anomalies.
Employee Fraud:
➧ Types: Employee fraud can encompass a range of activities, from theft of company assets to fraudulent expense claims.
➢ Data Analytics Application: Data analytics can monitor employee behavior within an organization's systems, flagging any unusual or unauthorized activities.
Vendor Fraud:
➧ Types: Vendor fraud includes inflated invoices, fictitious vendors, and kickbacks to employees.
➢ Data Analytics Application: Data analytics can cross-reference vendor data with invoices and purchase orders, detecting discrepancies that suggest fraud.
Data analytics plays a pivotal role in detecting and preventing various types of fraud. By leveraging the power of data, organizations can proactively identify anomalies, unusual patterns, and suspicious activities that may otherwise go unnoticed. With continuous advancements in data analytics techniques and technologies, the fight against fraud is becoming more sophisticated and effective, ultimately safeguarding the interests of individuals and businesses alike.
Auditing & Assurance
Table of contents
Syllabus
-
1.0
Introduction to Excel
- Microsoft excel key features
- Spreadsheet Interface
- Excel Formulas and Functions
- Data Analysis Tools
- keyboard shortcuts in Excel
- Conducting data analysis using data tables, pivot tables and other common functions
- Improving Financial Models with Advanced Formulas and Functions
-
2.0
Introduction to data analytics
-
3.0
Core application of data analytics
- Financial Accounting And Reporting
- Statement of Profit or Loss
- Statement of Financial Position
- Statement of Cash Flows
- Common Size Financial Statement
- Cross-Sectional Analysis
- Trend Analysis
- Analyse financial statements using ratios
- Graphs and Chats
- Prepare forecast financial statements under specified assumptions
- Carry out sensitivity analysis and scenario analysis on the forecast financial statements
- Data visualization and dash boards for reporting
- Financial Management
- Time value of money analysis for different types of cash flows
- Loan amortization schedules
- Project evaluation techniques using net present value - (NPV), internal rate of return (IRR)
- Carry out sensitivity analysis and scenario analysis in project evaluation
- Data visualisation and dashboards in financial management projects
4.0
Application of data analytics in specialised areas
- Management accounting
- Estimate cost of products (goods and services) using high-low and regression analysis method
- Estimate price, revenue and profit margins
- Carry out break-even analysis
- Budget preparation and analysis (including variances)
- Carry out sensitivity analysis and scenario analysis and prepare flexible budgets
- Auditing
- Analysis of trends in key financial statements components
- Carry out 3-way order matching
- Fraud detection
- Test controls (specifically segregation of duties) by identifying combinations of users involved in processing transactions
- Carry out audit sampling from large data set
- Model review and validation issues
- Taxation and public financial management
- Compute tax payable for individuals and companies
- Prepare wear and tear deduction schedules
- Analyse public sector financial statements using analytical tools
- Budget preparation and analysis (including variances)
- Analysis of both public debt and revenue in both county and national government
- Data visualisation and reporting in the public sector
5.0
Emerging issues in data analytics