4.0. Application of data analytics in specialised areas
4.2. Auditing
Model review and validation issues
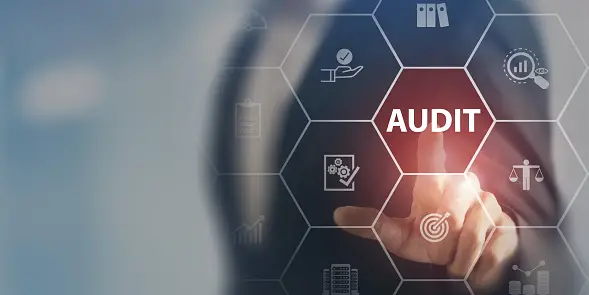
Application of Data Analytics in Auditing
What is model review
Model review is a critical step in the development and application of analytical models in various fields, including finance, data science, and auditing. It involves a comprehensive examination and evaluation of the model's structure, assumptions, data inputs, and methodology to ensure its accuracy, reliability, and relevance. In the context of auditing, model review plays a crucial role in assessing the effectiveness of analytical models used for tasks like risk assessment, fraud detection, and data analysis.
Related content:
4.2. Auditing
4.2.1. Analysis of trends in key financial statements components 4.2.2. Carry out 3-way order matching 4.2.3. Fraud detection 4.2.4. Test controls (specifically segregation of duties) by identifying combinations of users involved in processing transactions 4.2.5. Carry out audit sampling from large data set 4.2.6. Model review and validation issues
Model review and validation issues
In auditing, model review and validation are critical processes to ensure the accuracy and reliability of analytical models used for various audit procedures. Auditors often rely on models for tasks such as risk assessment, fraud detection, and data analysis. However, the effectiveness of these models hinges on their robustness and validity. During the review phase, auditors meticulously assess the model's design, data inputs, and underlying assumptions. They scrutinize whether the model aligns with the specific audit objectives and industry standards. Validation, on the other hand, involves testing the model's performance against historical data or real-world scenarios to confirm its predictive power and relevance. These processes are essential safeguards to prevent errors, biases, or misinterpretations in audit results, ensuring that auditors make informed decisions based on trustworthy data-driven insights.
Application of Data Analytics in Model Review and Validation in Auditing
In today's data-driven auditing landscape, the application of data analytics plays a pivotal role in enhancing the effectiveness and accuracy of model review and validation processes. Auditors increasingly rely on analytical models for risk assessment, fraud detection, and performance evaluation. Here, we delve into the ways data analytics is transforming the model review and validation procedures in auditing:
Enhanced Data Scrutiny:
Data analytics tools empower auditors to conduct more extensive and precise data scrutiny. They can analyze large datasets with speed and accuracy, identifying anomalies, outliers, and data quality issues that might affect the model's performance.
Automated Testing:
Data analytics enables automated testing of models against historical data, ensuring that models remain valid over time. This automation reduces the risk of errors and provides auditors with a continuous monitoring mechanism.
Scenario Analysis:
Auditors can use data analytics to perform scenario analysis, testing how different inputs and assumptions impact the model's outputs. This allows for a more comprehensive understanding of a model's behavior and its sensitivity to various factors.
Pattern Recognition:
Data analytics tools are adept at recognizing complex patterns within data. Auditors can leverage these capabilities to identify unusual patterns or trends that might indicate fraud or irregularities in financial data.
Machine Learning for Model Improvement:
Machine learning algorithms can be employed to continuously refine and improve models based on real-world data. This adaptive approach helps models remain relevant in dynamic audit environments.
Risk Assessment:
Data analytics assists auditors in conducting a more thorough risk assessment. They can use historical data and predictive analytics to identify areas of higher risk, focusing their efforts on these critical areas during the audit.
Efficiency Gains:
Data analytics streamlines the review process by automating repetitive tasks, allowing auditors to focus on higher-value activities like interpreting results and making informed decisions.
Documentation and Transparency:
Data analytics tools provide an audit trail of data transformations and model changes, enhancing transparency and accountability in the review and validation process.
Continuous Monitoring:
With data analytics, auditors can set up continuous monitoring systems, ensuring that models are regularly validated and updated as new data becomes available.
Interdisciplinary Collaboration:
Data analytics encourages collaboration between auditors and data scientists or data analysts. This interdisciplinary approach brings diverse expertise to model review and validation, resulting in more robust outcomes.
The application of data analytics in model review and validation issues in auditing is a transformative force. It enables auditors to not only identify risks and anomalies more effectively but also to adapt and improve their models continuously. This integration of data analytics fosters a data-driven audit environment, ensuring greater accuracy and reliability in financial reporting and compliance assessments.
Auditing & Assurance
Table of contents
Syllabus
-
1.0
Introduction to Excel
- Microsoft excel key features
- Spreadsheet Interface
- Excel Formulas and Functions
- Data Analysis Tools
- keyboard shortcuts in Excel
- Conducting data analysis using data tables, pivot tables and other common functions
- Improving Financial Models with Advanced Formulas and Functions
-
2.0
Introduction to data analytics
-
3.0
Core application of data analytics
- Financial Accounting And Reporting
- Statement of Profit or Loss
- Statement of Financial Position
- Statement of Cash Flows
- Common Size Financial Statement
- Cross-Sectional Analysis
- Trend Analysis
- Analyse financial statements using ratios
- Graphs and Chats
- Prepare forecast financial statements under specified assumptions
- Carry out sensitivity analysis and scenario analysis on the forecast financial statements
- Data visualization and dash boards for reporting
- Financial Management
- Time value of money analysis for different types of cash flows
- Loan amortization schedules
- Project evaluation techniques using net present value - (NPV), internal rate of return (IRR)
- Carry out sensitivity analysis and scenario analysis in project evaluation
- Data visualisation and dashboards in financial management projects
4.0
Application of data analytics in specialised areas
- Management accounting
- Estimate cost of products (goods and services) using high-low and regression analysis method
- Estimate price, revenue and profit margins
- Carry out break-even analysis
- Budget preparation and analysis (including variances)
- Carry out sensitivity analysis and scenario analysis and prepare flexible budgets
- Auditing
- Analysis of trends in key financial statements components
- Carry out 3-way order matching
- Fraud detection
- Test controls (specifically segregation of duties) by identifying combinations of users involved in processing transactions
- Carry out audit sampling from large data set
- Model review and validation issues
- Taxation and public financial management
- Compute tax payable for individuals and companies
- Prepare wear and tear deduction schedules
- Analyse public sector financial statements using analytical tools
- Budget preparation and analysis (including variances)
- Analysis of both public debt and revenue in both county and national government
- Data visualisation and reporting in the public sector
5.0
Emerging issues in data analytics