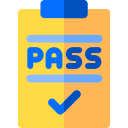
CPA
Foundation Leval
Quantitative Analysis November 2016
Suggested solutions
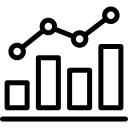
Revision Kit
➧ | Quantitative Analysis -September-2015-Pilot-Paper |
➧ | Quantitative Analysis-November-2015-Past-Paper |
➧ | Quantitative Analysis-May-2016-Past-paper |
➧ | Quantitative Analysis-November-2016-Past-Paper |
➧ | Quantitative Analysis-December-2017-Past-paper |
➧ | Quantitative Analysis-May-2017-Past-paper |
➧ | Quantitative Analysis-November-2018-Past-paper |
➧ | Quantitative Analysis-May-2018-Past-paper |
➧ | Quantitative Analysis-May-2019-Past-paper |
➧ | Quantitative Analysis-November-2019-Past-paper |
➧ | Quantitative Analysis-November-2020-Past-paper |
➧ | Quantitative Analysis-December-2021-Past-paper |
➧ | Quantitative Analysis-April-2021-Past-paper |
➧ | Quantitative Analysis-August-2021-Past-paper |
QUESTION 1(a)
Explanation: The constraints in a linear programming problem define a region in the solution space where valid solutions must lie. If this region is empty, meaning there is no point that satisfies all the constraints simultaneously, the problem is considered infeasible. Infeasibility could result from contradictory or incompatible constraints.
Definition: Unboundedness occurs when there is no finite optimal solution to the linear programming problem. In other words, the objective function can be improved indefinitely without reaching an optimal solution.
Explanation: Linear programming aims to optimize (maximize or minimize) a linear objective function subject to linear constraints. If there is no upper or lower limit on the objective function within the feasible region, the problem is considered unbounded. This situation might arise when the feasible region extends infinitely in one or more directions, and the objective function can improve indefinitely without reaching an optimal value.
Definition: Alternate optimality, also known as degeneracy, occurs when there are multiple optimal solutions to the linear programming problem.
Explanation: Ideally, a linear programming problem has a unique optimal solution. However, in some cases, there might be more than one combination of decision variables that yield the same optimal value for the objective function. These different solutions are considered alternate optimal solutions. Degeneracy can occur when the feasible region has redundant constraints or when the objective function is parallel to one of the constraint boundaries.
QUESTION 1(b)
QUESTION 1(c)
College | No. of students after advert | Pre advert students | d | (d-d̄)2 |
1 | 170 | 165 | 5 | 2.25 |
2 | 141 | 140 | 1 | 6.25 |
3 | 142 | 143 | -1 | 20.25 |
4 | 167 | 160 | 7 | 12.25 |
5 | 168 | 162 | 6 | 6.25 |
6 | 157 | 154 | 3 | 0.25 |
21 | 47.5 |
QUESTION 2(a)
Markov analysis is used to model customer behavior, predicting transitions between different states of loyalty or engagement. This helps in designing effective customer retention strategies.
Markov models analyze and predict changes in financial states of investment portfolios, assisting in risk assessment, investment decisions, and asset allocation strategies.
Markov analysis can model the transitions between different states in a supply chain, optimizing supply chain efficiency, reducing bottlenecks, and improving overall performance.
Markov models can be applied to analyze and predict transitions between different quality states of products during production. This helps in identifying potential defects and improving overall product quality.
Markov analysis is applied to project management to model the progression of projects through different phases or states. This helps in predicting project completion times and optimizing project schedules.
Markov models can be employed to analyze customer journeys through the marketing and sales funnel, optimizing marketing strategies and improving the sales process.
Markov analysis is used to model transitions between different risk states or insurance coverage states, aiding in pricing policies, assessing risk exposure, and optimizing insurance product offerings.
Markov models are applied in credit scoring to predict transitions between different credit risk states over time. This helps in assessing creditworthiness and optimizing lending practices.
Markov analysis is applicable in healthcare for modeling patient transitions between different health states, aiding in healthcare planning, resource allocation, and optimizing patient care.
QUESTION 2(b)
QUESTION 2(c)
QUESTION 3(a)
QUESTION 3(b)
Y | 4CMT | 4CMTA | CMACT | Y/T | Y-T |
250 | |||||
200 | 930 | 232.5 | |||
180 | 1010 | 252.5 | 242.5 | 0.742 | 62.5 |
300 | 1090 | 272.5 | 262.5 | 1.143 | 37.5 |
330 | 1170 | 292.5 | 282.5 | 1.168 | 47.5 |
280 | 1250 | 312.5 | 302.5 | 0.926 | -22.5 |
260 | 1330 | 332.5 | 322.5 | 0.806 | -62.5 |
380 | 1420 | 355 | 343.75 | 1.105 | 36.25 |
410 | 1,500 | 375 | 365 | 1.123 | 45 |
370 | 1580 | 395 | 365 | 0.961 | -15 |
340 | 1648 | 412 | 403.5 | 0.843 | -63.5 |
460 | |||||
478 |
Year | Q1 | Q2 | Q3 | Q4 | |
2012 | 0.742 | 1.143 | |||
2013 | 1.168 | 0.926 | 0.806 | 1.105 | |
2014 | 1.123 | 0.961 | 0.843 | ||
2015 | = | ||||
Total | 2.291 | 1.887 | 2.391 | 2.248 | |
Average | 1.146 | 0.994 | 0.797 | 1.124 | 0.4011 |
Year | Q1 | Q2 | Q3 | Q4 | |
2012 | -62.50 | 37.50 | |||
2013 | 47.50 | -22.50 | -62.50 | 36.25 | |
2014 | 45.00 | -15.00 | -63.50 | ||
Total | 92.50 | -37.50 | -188.50 | -73.75 | |
Average | 46.25 | -18.75 | -62.83 | 36.88 | 1.55 |
Adjustment factor | -0.39 | -0.39 | -0.39 | -0.39 | -1.55 |
ASI | 45.86 | -19.14 | -63.22 | 36.49 |
Year | Quater | Y | ASI | Deseasonalised data |
2012 | 1 | 250 | 45.86 | 204.14 |
2 | 200 | -19.14 | 219.14 | |
3 | 180 | -63.22 | 243.22 | |
4 | 300 | 36.49 | 263.51 | |
2013 | 1 | 330 | 45.86 | 284.14 |
2 | 280 | -19.14 | 299.14 | |
3 | 260 | -63.22 | 323.22 | |
4 | 380 | -63.22 | 323.22 | |
2014 | 1 | 410 | 45.86 | 364.14 |
2 | 370 | -19.14 | 389.14 | |
3 | 340 | -63.22 | 403.22 | |
4 | 460 | 36.49 | 423.51 | |
2015 | 1 | 478 | 45.86 | 432.14 |
QUESTION 4(a)
Linear programming assumes additivity and proportionality, which may not accurately represent complex real-world relationships.
Linear programming assumes that all parameters, coefficients, and data are known with certainty, neglecting uncertainties and variability.
Linear programming assumes continuous decision variables, while in reality, some decisions may be discrete.
Linear programming models are generally static, not accounting for dynamic or time-dependent changes over time.
Linear programming is designed for linear objective functions, limiting its applicability in situations with non-linear objectives.
Linear programming solutions can be sensitive to changes in coefficients and parameters, impacting the robustness of the model.
Linear programming does not inherently account for the time value of money, crucial in many decision-making scenarios.
Linear programming assumes independence between decision variables, which may not hold true in real-world interdependent scenarios.
Solving large-scale linear programming problems can be computationally demanding, especially with a large number of variables and constraints.
Linear programming is primarily quantitative and may struggle to handle qualitative or non-quantifiable factors effectively.
QUESTION 4(b)
Decision Rule: Choose the alternative with the highest possible maximum payoff.
Description: Select the alternative that maximizes the minimum possible payoff.
Decision Rule: Choose the alternative with the highest minimum payoff.
Description: Focuses on minimizing the regret associated with each decision.
Decision Rule: Minimize the maximum regret, where regret is the difference between the payoff of the chosen alternative and the best possible payoff for each state of nature.
Description: A compromise between optimism and pessimism, using an optimism coefficient (α) to weigh the minimum and maximum payoffs.
Decision Rule: Calculate the weighted average for each alternative and choose the one with the highest value.
Description: Calculates the average payoff for each alternative, considering the probabilities of different states of nature.
Decision Rule: Choose the alternative with the highest expected monetary value.
Description: Measures the average opportunity loss for each alternative.
Decision Rule: Choose the alternative with the lowest expected opportunity loss.
Description: Measures the value of acquiring additional information to eliminate uncertainty.
Decision Rule: Compare the expected payoff with perfect information to the expected payoff without additional information.
Description: Utilizes a graphical representation of decision alternatives, probabilities, and payoffs to analyze complex decision scenarios.
Decision Rule: Evaluate decision nodes, probability nodes, and terminal nodes to identify the optimal decision path.
Description: Applies financial options concepts to evaluate the value of flexibility in decision-making.
Decision Rule: Incorporate the value of deferring, expanding, or abandoning a decision based on changing circumstances.
Description: Applies Bayesian probability to update beliefs and make decisions as new information becomes available.
Decision Rule: Update probabilities based on prior knowledge and new evidence to revise decision choices.
QUESTION 4(c)
QUESTION 4(d)
QUESTION 5(a)
Limitation: Many game models assume that players have complete information about the game, including the payoffs and strategies of all players. In practice, information may be incomplete or asymmetric, leading to different outcomes.
Limitation: Game theory often models situations as one-shot games or in discrete time steps. In dynamic and evolving environments, where interactions occur continuously over time, traditional game theory may not capture the complexity of strategic decisions.
Limitation: Game theory becomes more complex as the number of players increases. Analyzing games with a large number of players becomes computationally challenging, and finding equilibrium solutions may become impractical.
Limitation: Game theory assumes that players have common knowledge of the rules, strategies, and payoffs. Achieving common knowledge in real-world scenarios can be challenging, and assumptions of common knowledge may not hold.
Limitation: While Nash equilibrium is a central concept in game theory, not all games have unique equilibria, and some games may have multiple equilibria. The predictive power of Nash equilibrium relies on the uniqueness and stability of equilibria.
Limitation: In certain situations, players may find it difficult to randomize their strategies, especially if they have a strong preference for one particular strategy. The assumption of players employing mixed strategies may not always hold.
Limitation: Traditional game theory assumes that players act independently and do not cooperate. In reality, cooperation and collusion among players are common, and game theory might not fully capture cooperative strategies.
Limitation: In extensive-form games, players are expected to make sequentially rational decisions. However, in situations of imperfect information or changing circumstances, players may deviate from their intended strategies.
Limitation: Game theory often overlooks behavioral aspects, such as emotions, trust, and social norms, which can significantly influence decision-making in strategic interactions.
Limitation: Game theory assumes fixed strategies, but in reality, players may adapt their strategies based on feedback and learning from previous interactions. Dynamic and adaptive behavior is not always adequately captured.
Limitation: Game theory may not account for external factors, such as regulatory changes, economic shocks, or technological advancements, that can impact the strategies and payoffs of players.
QUESTION 5(b)
Activity | Expected time |
A | [1.5 + (4 x 2) + 2.5] ÷ 6 = 2 |
B | (2 + 2.5 x 4 + 6 ) ÷ 6 = 3 |
C | (1 + 2 x 4 + 3 ) ÷ 6 = 2 |
D | (1.5 + 2 x 4 + 2.5) ÷ 6 = 2 |
E | (0.5 + 1 x 4 + 1.5) ÷ 6 = 1 |
F | (1 + 2 x 4 + 3) ÷ 6 = 2 |
G | (3 + 3.5 x 4 + 7) ÷ 6 = 4 |
H | (3 + 4 x 4 + 5) ÷ 6 = 4 |
I | (1.5 + 2 x 4 + 2.5) ÷ 6 = 2 |
➦ | Economics-September-2015-Pilot-Paper |
➦ | Economics-November-2015-Past-Paper |
➦ | Economics-May-2016-Past-paper |
➦ | Economics-November-2016-Past-Paper |
➦ | Economics-November-2017-Past-paper |
➦ | Economics-May-2017-Past-paper |
➦ | Economics-November-2018-Past-paper |
➦ | Economics-May-2018-Past-paper |
➦ | Economics-May-2019-Past-paper |
➦ | Economics-November-2019-Past-paper |
➦ | Economics-November-2020-Past-paper |
➦ | Economics-December-2021-Past-paper |
➦ | Economics-April-2021-Past-paper |
➦ | Economics-August-2021-Past-paper |
➧ | Introduction to Law and Governance-September-2015-Pilot-Paper |
➧ | Introduction to Law and Governance-November-2015-Past-Paper |
➧ | Introduction to Law and Governance-May-2016-Past-paper |
➧ | Introduction to Law and Governance-November-2016-Past-Paper |
➧ | Introduction to Law and Governance-May-2017-Past-paper |
➧ | Introduction to Law and Governance-November-2017-Past-Paper |
➧ | Introduction to Law and Governance-November-2018-Past-paper |
➧ | Introduction to Law and Governance-May-2018-Past-paper |
➧ | Introduction to Law and Governance-May-2019-Past-paper |
➧ | Introduction to Law and Governance-November-2019-Past-paper |
➧ | Introduction to Law and Governance-November-2020-Past-paper |
➧ | Introduction to Law and Governance-December-2021-Past-paper |
➧ | Introduction to Law and Governance-April-2021-Past-paper |
➧ | Introduction to Law and Governance-August-2021-Past-paper |
➧ | Financial Accounting -September-2015-Pilot-Paper |
➧ | Financial Accounting -November-2015-Past-Paper |
➧ | Financial Accounting -May-2016-Past-paper |
➧ | Financial Accounting-November-2016-Past-Paper |
➧ | Financial Accounting-November-2017-Past-paper |
➧ | Financial Accounting-May-2017-Past-paper |
➧ | Financial Accounting-November-2018-Past-paper |
➧ | Financial Accounting-May-2018-Past-paper |
➧ | Financial Accounting-May-2019-Past-paper |
➧ | Financial Accounting-November-2019-Past-paper |
➧ | Financial Accounting-November-2020-Past-paper |
➧ | Financial Accounting-December-2021-Past-paper |
➧ | Financial Accounting-April-2021-Past-paper |
➧ | Financial Accounting-August-2021-Past-paper |
CPA past papers with answers